Unlocking the Potential of Machine Learning Data Labeling
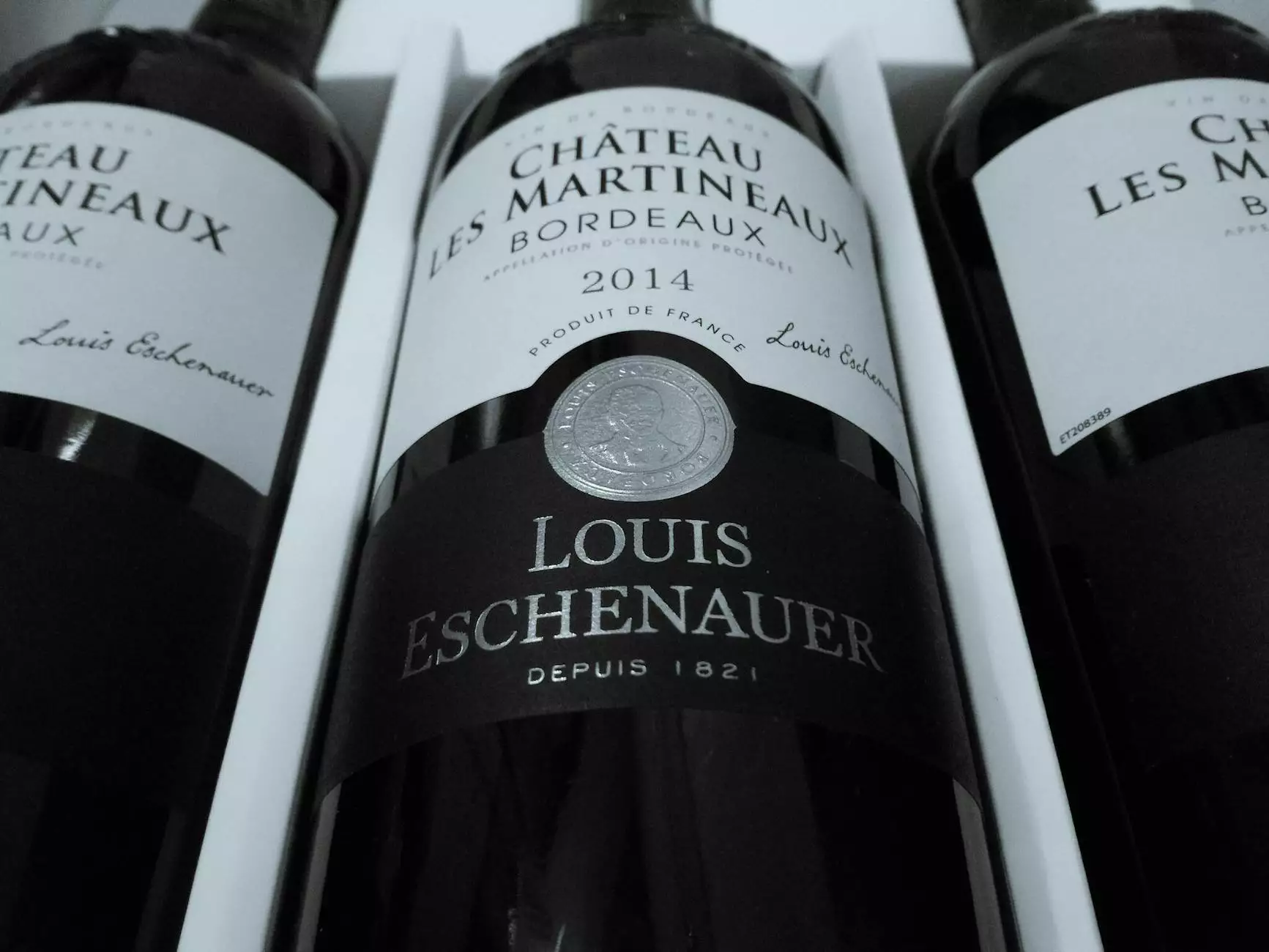
Understanding Machine Learning Data Labeling
In the age of artificial intelligence (AI), machine learning data labeling has emerged as a critical component in developing intelligent systems. This process involves tagging or annotating datasets to provide context and meaning, enabling machine learning algorithms to learn from them. The success of an AI model often hinges on the quality and accuracy of the data it is trained on, making data labeling an integral part of the machine learning pipeline.
The Importance of Data Annotation in AI Development
Data annotation serves as the bridge between raw data and machine learning insights. High-quality annotations ensure that models not only understand data but also make predictions with remarkable accuracy. Here are several reasons why data annotation is vital:
- Enhances Model Accuracy: Well-labeled data provides precise input for models, significantly improving their predictive capabilities.
- Facilitates Data Understanding: Annotation supplies essential information about the dataset, fostering better model training and evaluation.
- Customizes Solutions: Different industries have distinct needs. Tailored data labeling helps develop specialized AI solutions.
- Increases Scalability: Automated and semi-automated labeling processes can handle vast datasets, which is crucial for training large models.
Exploring Data Annotation Tools
Choosing the right data annotation tool can significantly enhance the efficiency of the machine learning data labeling process. Various tools are available, each offering unique features designed to streamline annotation tasks. Some popular tools include:
- Keylabs.ai: Offers a comprehensive platform for data annotation, combining accuracy with speed. It is particularly known for its user-friendly interface and tools tailored for specific use cases.
- Labelbox: A collaborative training data platform that provides flexible labeling for diverse datasets, ideal for teams working on complex projects.
- Amazon SageMaker Ground Truth: Integrates human and machine labeling to produce high-quality training datasets quickly and efficiently.
- SuperAnnotate: Specialized in computer vision tasks, it provides an intuitive platform with tools for image and video annotation.
The Data Annotation Platform: A Game Changer
A robust data annotation platform can transform how organizations approach AI development. By centralizing data labeling processes, these platforms ensure consistency and quality. Here are some key features that define an effective data annotation platform:
- Collaboration Tools: Facilitate teamwork and improved communication among data scientists, annotators, and project managers.
- Automation Capabilities: Accelerate the labeling process through artificial intelligence and machine learning technologies that assist human annotators.
- Quality Assurance: Implement verification processes that ensure the accuracy and reliability of labeled data.
- Scalability: Easily manage increasing volumes of data as organizations grow and their needs evolve.
Best Practices for Machine Learning Data Labeling
Engaging in best practices during the machine learning data labeling process can lead to outstanding results. Organizations should consider the following:
- Define Clear Objectives: Understand what you aim to achieve with machine learning. Clear objectives guide the annotation process and ensure data relevance.
- Invest in Training: Annotators should receive comprehensive training regarding the specific requirements and expectations for each project.
- Regular Quality Checks: Continually monitor the quality of annotations through audits and reviews to maintain high standards.
- Utilize Feedback Loops: Create channels for annotators to provide feedback on the labeling guidelines or challenges encountered during the annotation process.
- Leverage Technology: Incorporate AI-assisted tools that can speed up the process while maintaining quality.
Challenges in Data Labeling
Despite its importance, machine learning data labeling is not without challenges. Organizations often face hurdles that can hinder the efficiency and accuracy of the data annotation process:
- Subjectivity: Different annotators may interpret labeling criteria differently, leading to inconsistencies.
- Scalability Issues: As project sizes grow, managing and coordinating a large team of annotators can become complicated.
- Time-Consuming: Manual annotation can be labor-intensive and time-consuming, often causing project delays.
- Data Privacy: Organizations must ensure compliance with data protection regulations while handling sensitive data.
Embracing the Future of Data Annotation
As technology evolves, so does the field of data annotation. Here are emerging trends that are shaping the future of machine learning data labeling:
1. Automation and Artificial Intelligence
Machine learning algorithms are being harnessed to automate portions of the data labeling process. By utilizing AI, organizations can reduce manual effort and increase efficiency. AI can assist with:
- Pre-labeling data, providing a starting point for human annotators.
- Identifying anomalies or incorrect labels within the dataset.
- Streamlining repetitive tasks, allowing annotators to focus on complex labeling.
2. Enhanced Collaboration Tools
Future data annotation platforms will likely emphasize collaboration, offering integrated communication tools that keep annotators, data scientists, and project managers connected. Improved tools enable:
- Real-time feedback and adjustments to labeling guidelines.
- Shared documentation and training resources to foster continuous learning.
- Project tracking to maintain deadlines and manage resources effectively.
3. Focus on Ethical Data Practices
As AI becomes more pervasive, the importance of ethical data practices cannot be overstated. Organizations must prioritize fairness, accountability, and bias mitigation in their data labeling efforts. Relevant approaches include:
- Conducting regular audits of the labeled data to detect and correct biases.
- Ensuring diverse annotation teams to capture different perspectives.
- Adopting guidelines that promote ethical data use throughout the machine learning lifecycle.
The Role of Keylabs.ai in Data Annotation
Keylabs.ai stands at the forefront of the machine learning data labeling revolution, providing cutting-edge data annotation tools and platforms. The company focuses on:
1. Specialized Annotation Services
Keylabs.ai offers tailored solutions for diverse industries, from healthcare to autonomous vehicles. Their annotation services ensure that:
- The data is accurately labeled according to industry-specific guidelines, enhancing model performance.
- Clients receive comprehensive support throughout the annotation process.
2. Advanced Technology Integration
The integration of advanced technologies allows Keylabs.ai to leverage automation and AI to assist with data labeling. The outcomes result in:
- Increased efficiency and speed of the annotation process.
- High-quality labeled data that aligns with client goals and benchmarks.
3. Committed Quality Assurance
Keylabs.ai prioritizes quality through ongoing checks and feedback mechanisms, ensuring that:
- All labeled data meets industry standards and client specifications.
- Continuous improvement in the annotation process based on client feedback.
Conclusion: The Future of Machine Learning Data Labeling
As machine learning continues to grow, the role of machine learning data labeling will only increase in significance. Organizations that invest in high-quality data annotation will be well-positioned to harness the full potential of AI technologies. With platforms like Keylabs.ai paving the way, businesses can look forward to a future filled with innovative solutions and remarkable advancements in artificial intelligence.
In a data-driven world, prioritizing effective data labeling is not just an option; it's a necessity for success in the computational landscape.